13th IAPR International Workshop on Document Analysis Systems
24-27 April
Vienna, Austria
About
DAS 2018 is the 13th edition of the 100% participation single-track workshop focusing on system-level issues and approaches in document analysis and recognition. The workshop comprises invited speaker presentations, oral, poster, tutorial sessions, as well as working group discussions. The Conference Publishing Services (CPS) will publish the workshop proceedings.
DAS 2018 will be organized at TU Wien (Vienna University of Technology), in the heart of Vienna’s city center, which places the attendees within walking distance of a large variety of world-famous historical and cultural attractions.
DAS 2018 will include both long and short papers, posters and demonstrations of working or prototype systems. All submissions will undergo a rigorous single blind review process with a minimum of 3 reviews considering the originality of work, the quality of research or analysis of experience, the relevance to document analysis systems, and quality of presentation.
Recap
Of the 131 submissions received in an open call for papers, 77 were accepted for presentation at the workshop (58.8%). Of these, 32 papers were designated for oral presentation (24.4%) and 45 for poster presentation (34.35%) after a rigorous review process directed by the four Program Chairs (Basilis Gatos, Koichi Kise, Dan Lopresti and Jean-Marc Ogier). All submissions received at least 2 reviews from 67 members of the program committee; most papers received 3 reviews. In addition, 12 short papers describing emerging ideas and work in progress were also accepted for poster presentation.
The IAPR Nakano Best Paper Award was presented to the best overall paper presented at the workshop: “Word Spotting and Recognition using Deep Embedding” by Praveen Krishnan, Kartik Dutta and C. V. Jawahar during the workshop banquet. The IAPR Best Student Paper Award was presented to Daniel Stromer, Vincent Christlein, Andreas Maier, Xiaolin Huang, Patrick Zippert, Eric Helmecke and Tino Hausotte for their paper “Non-Destructive Digitization of Soiled Historical Chinese Bamboo Scrolls”.
Program
Tutorials will be on Tuesday, April 24th. The welcome takes place on Tuesday 7pm at TUtheSky. You can download the .
Time | Wednesday, April 25th | Thursday, April 26th | Friday, April 27th |
---|---|---|---|
08:00 | Registration | ||
08:30 | Registration | Registration | |
09:00 | Opening | Oral Session 5 Scene Text Detection and Recognition |
Keynote 2 Rolf Ingold |
09:30 | Keynote 1 Lawrence O'Gorman |
||
10:00 | Oral Session 7 Document Understanding and Table Recognition |
||
10:30 | Coffee Break | Coffee Break | |
11:00 | Oral Session 1 Word Spotting |
Discussion groups | Coffee Break |
11:30 | Oral Session 8 Graphics Recognition |
||
12:00 | Oral Session 2 Handwriting Recognition |
||
12:30 | |||
13:00 | Lunch | Lunch | Lunch |
13:30 | |||
14:00 | |||
14:30 | Oral Session 3 Historical Document Analysis |
Oral Session 6 Document Analysis Applications |
Oral Session 9 Forensic Document Analysis |
15:00 | |||
15:30 | |||
16:00 | Teasers | Teasers | Discussion group reports |
16:30 | Poster Session 1 | Poster Session 2 | |
17:00 | Conclusion and awards | ||
17:30 |
Oral Session 4 |
||
18:00 | Social Event | ||
18:30 | |||
19:00 | Workshop banquet |
Please let us know which tutorials you plan to attend. (Click the images for more details)
From Digital Libraries to Kind Cameras
Making Sense of Multimedia Signals While (Unsuccessfully) Avoiding Security
Wednesday, April 25th 09:30 - 10:30
Lawrence O'Gorman, Nokia Bell Labs, Murray Hill, NJ USA
In the last 30 years, we have made great strides in computer analysis and understanding of signals from images to documents to video. In this talk, I describe projects whose initial objective was a useful and disruptive - and sometimes fun - multimedia recognition system, but for which security issues were discovered that complicated design and usability.
The first project involves document layout analysis methods to facilitate one of the first digital libraries, Bell Labs RightPages. However, publishers would not offer material through the system until we developed watermarking methods to assert their ownership. The second project is a voice-only system for healthcare workers to enable hands-free communications. But the system was impractical without authentication: how do you securely speak a password? The third project was for security purposes only, to design a counterfeit-resistant photo-ID card that can be retrofited to current non-secure cards, printed on paper, and even duplicated. We accomplished this in the early days of public key cryptography. Finally I will describe current work in "Kind Cameras", for which video analytics methods have been developed to extend past security cameras to interactive cameras for fun and art. The slides are available .
Lawrence O'Gorman is a Fellow at Nokia Bell Labs Research in Murray Hill, NJ. He works in the areas of video analysis and multimedia signal processing. Previously he was Chief Scientist at Veridicom, a biometric company, spun off from Lucent, and before that a Distinguished Member of Technical Staff at Bell Labs. He has taught in the area of multimedia security at Cooper Union and NYU/Poly. His video analytics work is the basis of the "Pixelpalooza" exhibit at the Liberty Science Center in New Jersey, and other public art and game exhibits.
He has published over 70 technical papers, 8 book chapters, holds over 25 patents, and is co-author of the books, "Practical Algorithms for Image Analysis" published by Cambridge University Press, and "Document Image Processing" published by IEEE Press. He is a Fellow of the IEEE and of the IAPR. In 1996, he won the Best Industrial Paper Award at the ICPR and an R&D 100 Award for one of "the top 100 innovative technologies of that year." He has been on the editorial boards of 4 journals, and has served on US government committees to NIST, NSF, NIJ, and NAE, and to France's INRIA.
He received the B.A.Sc., M.S., and Ph.D. degrees in electrical engineering from the University of Ottawa, University of Washington, and Carnegie Mellon University respectively.
Lessons from 10 Years of Experience on Historical Document Analysis
Friday, April 27th 09:00 - 10:00
Rolf Ingold, DIVA Group, University of Fribourg, Switzerland
Libraries and archives all around the world continuously increase their efforts in digitizing historical manuscripts. Integrating such manuscripts into digital libraries requires meaningful information for indexing. To support the extraction of the needed meta-information or to provide full-text transcription, advanced pattern recognition and machine learning methods are required. This talk will describe the outcome of a series of "HisDoc" research projects funded by the Swiss National Foundation, covering pioneering attempts to study the whole processing chain from layout analysis to information retrieval of historical manuscripts, including script analysis, word spotting and handwriting recognition. This description will be complemented with an overview of other related research projects, in order to convey the current state of the art in the field and outline future trends.
Rolf Ingold is full professor of computer science at the University of Fribourg, in Switzerland. He graduated in mathematics and received a PhD degree in Computer Science from the Swiss Federal Institute of Technology Lausanne (EPFL). He is an international expert in the field of document analysis and modelling. Since 2008, he is Chair of the Swiss Pattern Recognition Association and member of the Governing Board of the International Association for Pattern Recognition (IAPR). At National level, he was highly involved in the Swiss National Center of Competence on Interactive Multimodal Information Management. He is also member of the Swiss Academy of Science and Technology.
His expertise covers several aspects of multimodal signal processing, pattern recognition, and machine learning, with applications in image and sound analysis, biometry, and gesture recognition. During the last fifteen years, he concentrated his research on historical document analysis and cultural heritage preservation. Since 2009, he is leading a series of research projects funded by the Swiss National Foundation on historical document analysis. In 2011, he received the James A. Lindner price awarded by the International Association of Sound and Audiovisual Archives (IASA) for "VisualAudio", a solution to recover sound from degraded sound tracks.
Introduction to DL in Theory and Practice
Tuesday, April 24th 09:30 - 10:30
René Donner, contextflow, Austria
In this tutorial we will look at the basics of Deep Learning (DL), the reasons for its recent success and which tasks it can be applied to. The participants will also get an overview over the current landscape of DL frameworks, teaching resources and how to get started with DL in their own work. The slides are available here: .
With a background in electrical engineering René has worked for 8 years at the Medical University Vienna as a researcher in computer vision, focusing on anatomical structure localization and content based image retrieval. He is now CTO at contextflow, applying deep learning to large scale medical image data and developing smart tools to aid radiologists in their challenging tasks.
Keyword Spotting for Large-Scale Indexing and Search in massive Document collections
Tuesday, April 24th 11:00 - 13:30
Alejandro H. Toselli, Emilio Granell, Joan Puigcerver
Universitat Politècnica de València
Libraries, archives and other cultural institutions all over the world are making accessible large amounts of digital handwriting documents most of which lacks transcripts. This fact has motivated the development of handwritten processing technologies; namely automatic/assisted handwritten text recognition (HTR) and keyword spotting (KWS), to provide access to the images textual contents. In this line, this tutorial is intended to present from theoretical and practical perspectives, a comprehensive insight of two state-of-the-art technologies, based on Deep Learning approaches: "Laia: A deep learning toolkit for HTR, based on Torch and Kaldi" and "Keyword Spotting for Large-Scale Indexing and Search in massive Document collections". Laia, besides obtaining highly accurate recognized transcripts, produces primary structures called "word-graphs" which are subsequently used by the KWS techniques to produce problematically-sound word confidence scores for indexing. The proposed tutorial is planed to be given in 2 hours. It is assumed that the students will have a prior general knowledge on Pattern Recognition and some experience in Handwriting Text Recognition and Deep Learning.
Slides are available here.
Alejandro H. Toselli received the M.S. degree in electrical engineering from Universidad Nacional de Tucumán (Argentina) in 1997 and the Ph.D. degree in Computer Science from Universitat Politècnica de València (Spain) in 2004. He did a post-doc at the "Institut de Recherche en Informatique et Systémes Aléatoires" (IRISA), France. His current research interest lies in the subject of Computer Assisted and Interaction in Pattern Recognition Systems: Handwritten Text Recognition and Keyword Spotting Applications. He has teaching experience in imparting different tutorial courses about the "Handwritten Text Recognition" topic.
Emilio Granell obtained his BSc in Telecommunications Engineering with the speciality in Sound and Image in 2006, his MSc degree in Artificial Intelligence, Pattern Recognition, and Digital Image in 2011, and his Ph.D. degree in Computer Science in 2017, all from Universitat Politècnica de València (UPV). Dr. Granell pertains to the Pattern Recognition and Human Language Technology (PRHLT) research center, where he develops his research on the topics of speech and handwriting recognition, dialogue systems, and interactive and multimodal systems. From 2010 he has participated in several research projects related with artificial intelligence, speech and handwriting recognition, and smart cities.
Joan Puigcerver is a PhD candidate in Computer Science at the Universitat Politècnica de València at the Pattern Recognition and Human Language Technology Research Center. He previously received the Engineer's degree in Computer Science (2012) and the Master's degree in Pattern Recognition and Artificial Intelligence (2014) from the same institution. He is broadly interested in statistical pattern recognition and machine learning, and its applications to computer vision, handwritten text recognition and keyword spotting. He is member of the IEEE Computer Society and the Spanish Society for Pattern Recognition and Image Analysis (AERFAI).
Deep Learning for Document Analysis, Text Recognition, and Language Modeling
Tuesday, April 24th 14:30 - 18:30
Thomas Breuel, NVIDIA Research, USA
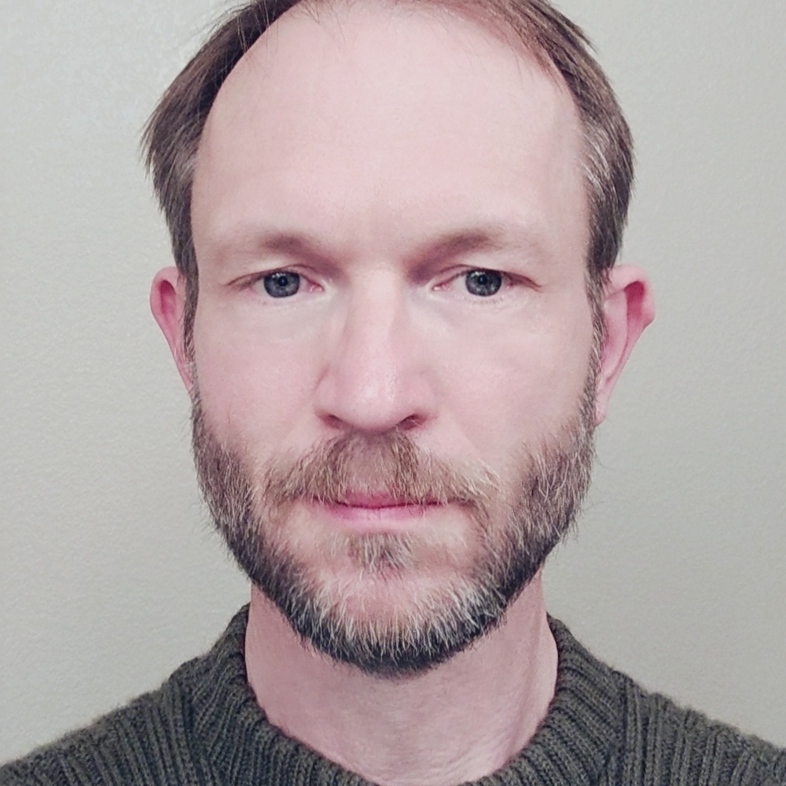
The tutorial will cover applications of deep learning to problems in document analysis:
- convolutional, one-dimensional, and multidimensional layers
- the relationship between filters and deep learning models
- different types of sequence models: LSTM, seq2seq, attention, CTC
- DL models for noise removal, upscaling, skew correction
- DL models for layout analysis and semantic segmentation
- DL models for OCR, handwriting recognition, text recognition
- DL models for language modeling and OCR post-correction
- preprocessing, scaling, and GPU-based computing
Thomas Breuel works on deep learning and its applications at NVIDIA Research. Before that, he was a researcher at Google Brain, IBM, and Xerox PARC. He was a professor of computer science and head of the Image Understanding and Pattern Recognition (IUPR) at the University of Kaiserslautern. He has published numerous papers in document analysis, computer vision, and machine learning and is a contributor to several open source projects in OCR, document analysis, and machine learning.
Reproducible Research in Document Image Analysis
Tuesday, April 24th 11:00 - 13:30
Marcel Würsch, Michele Alberti, Vinaychandran Pondenkandath, Marcus Liwicki
DIVA Group, University of Fribourg, Switzerland
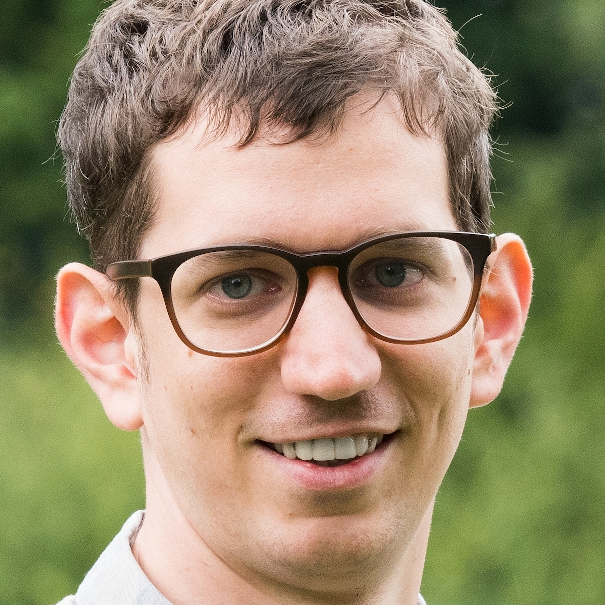
An important topic not only in Document Image Analysis but in Machine Learning in general is the reproducibility of scientific results. Many papers get published today where it is increasingly difficult for the reader to independently reproduce and verify the reported numbers, due to various reasons. In this tutorial we will provide introductions and hands on sessions on existing solutions. DeepDIVA a Deep Learning toolkit with a focus on creating reproducible experiments, and DIVAServices a Web Service framework providing access to DIA methods.
The slides are available online.
Marcel Würsch is a PhD student in the DIVA research group at the University of Fribourg. The main work of his dissertation is DIVAServices, a Web Service framework for providing Document Image Analysis methods as RESTful Web Services.
Word Spotting
Wednesday, April 25th 11:00 - 12:00
Handwriting Recognition
Wednesday, April 25th 12:00 - 13:00
Historical Document Analysis
Wednesday, April 25th 14:30 - 16:00
It utilizes deep convolutional nets (CNNs) for both, the actual extraction of baselines, as well as for a simple form of layout analysis in a pre-processing step. To the best of our knowledge it is the first CNN-based system for baseline extraction applying a U-net architecture and sliding window detection, profiting from a high local accuracy of the candidate lines extracted. Final baseline post-processing complements our approach, compensating for inaccuracies mainly due to missing context information during sliding window detection.
We experimentally evaluate the components of our system individually on the cBAD dataset. Moreover, we investigate how it generalizes to different data by means of the dataset used for the baseline extraction task of the ICDAR 2017 Competition on Layout Analysis for Challenging Medieval Manuscripts (HisDoc). A comparison with the results reported for HisDoc shows that it also outperforms the contestants of the latter.
Databases and Benchmarking
Wednesday, April 25th 17:30 - 18:30
Scene Text Detection and Recognition
Thursday, April 26th 9:00 - 10:30
The multi-level CC analysis allows the extraction of redundant text and non-text components at multiple binarization levels to minimize the loss of any potential text candidates. The features of the resulting raw text/non-text components of different granularity levels are learned via a CNN. Those two modules eliminate the need for complex ad-hoc preprocessing steps for finding initial candidates, and the need for hand-designed features to classify such candidates into text or non-text.
The components classified as text at different granularity levels, are grouped in a graph based on the overlap of their extended bounding boxes, then, the connected graph components are retained. This eliminates redundant text components and forms words or textlines.
When evaluated on the "Robust Reading Competition" dataset for natural scene images, our method achieved better detection results compared to state-of-the-art methods. In addition to its efficacy, our method can be easily adapted to detect multi-oriented or multi-lingual text as it operates at low level initial components, and it does not require such components to be characters.
detection method in both aspects of accuracy and efficiency, but it is still not very sensitive to the small text in natural scenes and often can not localize text regions precisely. To tackle these problems, we first present a Bidirectional Information Aggregation (BIA) architecture by effectively aggregating multi-scale feature maps to enhance local details and strengthen context information, making the detector not only work reliably on the small text, but also predict more precise boxes for texts. This architecture also results in a single classifier network, which allows our model to be trained much faster and easily with better generalization power. Then, we propose to use symmetrical feature maps for feature extraction both in the training and test stages for further improving the performance on the small text. To further promote precise predicting boxes, we present a statistical grouping method that operates on the training set bounding boxes to generate adaptive aspect ratios for default boxes. Finally, our model not only outperforms the TextBoxes without much time overhead, but also provides promising performance compared to the recent state-of-the-art methods on the ICDAR 2011 and 2013 database.
Document Analysis Applications
Thursday, April 26th 14:30 - 16:00
Since the last years, with the development of low-cost eye trackers, the technology is now accessible for many people, which will allow using data mining and machine learning algorithms for the mutual analysis of documents and readers.
Document Understanding and Table Recognition
Friday, April 27th 10:00 - 11:00
Graphics Recognition
Friday, April 27th 11:30 - 13:00
Forensic Document Analysis
Friday, April 27th 14:30 - 16:00
In this work, we compare the established VLAD encoding with triangulation embedding. We further investigate generalized max pooling as an alternative to sum pooling and the impact of decorrelation and Exemplar SVMs. With these techniques, we set new standards on two publicly available datasets (ICDAR13, KHATT).
In this work, automated morph detection algorithms based on general purpose pattern recognition algorithms are benchmarked for two scenarios relevant in the context of fraud detection for electronic travel documents, i.e. single image (no-reference) and image pair (differential) morph detection. In the latter scenario a trusted live capture from an authentication attempt serves as additional source of information and, hence, the difference between features obtained from this bona fide face image and a potential morph can be estimated. A dataset of 2,206 ICAO compliant bona fide face images of the FRGCv2 face database is used to automatically generate 4,808 morphs. It is shown that in a differential scenario morph detectors which utilize a score level-based fusion of detection scores obtained from a single image and differences between image pairs generally outperform no-reference morph detectors with regard to the employed algorithms and used parameters. On average a relative improvement of more than 25% in terms of detection equal error rate is achieved.
Poster Session I (Wednesday 25th April, 16:00-17:30)
Tablets are being acquired from different sources requiring different methods for digitalization. Each representation is typically processed with its own tool-set. To homogenize these data sources, we introduce an unifying minimal wedge constellation description. For this representation, we develop similarity metrics based on the optimal assignment of wedge configurations.
We combine our wedge features with work on segmentation-free word spotting using part-structured models. The presented search and similarity facilities enable the development of advances linguistic tools for cuneiform sign indexing and spatial n-gram mining of signs.
Cremona (Italy). This data set is very complex, it contains only short writings (few words or text lines), faded or damaged areas, different supports (wood or paper), and various annotations added by the owners of this collection during the centuries. Experimental results is promising showing an accuracy greater than 90% using short texts both as training and as target.
The flow is a collection of consecutive scanned pages without explicit separation marks between documents. Our method is based on contextual and layout descriptors meant to specify the relationship between each pair of consecutive pages. The relationships are represented using vectors of features with boolean values indicating the presence or the absence of descriptors on concerned pages. The segmentation task therefore consists in classifying such vectors into continuities or breaks. The continuity class indicates that pages belong to the same document while the break class ends the ongoing document and starts a new one. The experimental part is based on a large collection of real administrative documents.
limits of the existing state-of-the-art methods such as the delay of the system efficiency. This is a concern in industrial context when we have only few samples of each document class. Based on this analysis, we propose a hybrid system combining incremental learning by means of tf-idf statistics and a-priori generic models. We report in the experimental section our results obtained with a large dataset of real invoices.
In this paper, we propose a recurrent neural network based algorithm using Grid Long Short-Term Memory cells for image binarization, as well as a pseudo F-Measure based weighted loss function. We evaluate the binarization and execution performance of our algorithm for different choices of footprint size, scale factor and loss function. Our experiments show a significant trade-off between binarization time and quality for different footprint sizes. However, we see no statistically significant difference when using different scale factors and only limited differences for different loss functions. Lastly, we compare the binarization performance of our approach with the best performing algorithm in the 2016 handwritten document image binarization contest and show that both algorithms perform equally well.
In this paper, we propose an approach to digitize track layouts (semi-)automatically. We use fingerprint recognition techniques to digitize manually created track plans efficiently. At first, we detect tracks by detecting line endings and bifurcations. Secondly, we eliminate false candidates and irregularities. Finally, we translate the resulting graph into an interchangeable format RailML.
We evaluate our method by comparing our results with different track plans. Our results indicate that the proposed method is a promising candidate, reducing the effort of digitization.
Although, there are many on-line web services to make new logos, they have limited designs and duplicates could be made.
We propose using neural style transfer with clip art and text for the creation of new and genuine logos.
We introduce a new loss function based on distance transform of input image, which allows the preservation of the silhouettes of text and objects.
The proposed method contains style transfer to only a designated area.
We demonstrate the characteristics of proposed method.
Finally, we show the results of logo generation with various input images.
Poster Session II (Thursday 26th April, 16:00-17:30)
intensive work for many paleographers. Here we have presented an end to end semi automatic interactive text alignment system for historical document. OCRopus [14] is used for binarization and line segmentation of the historical document image. Text line segmentation followed by text alignment is done automatically by the system using ORB (Oriented Fast and Rotated Brief) local image feature descriptors. ORB features are matched by KNN. The system provides an interactive user interface for rectifying wrong text segmentation and text alignment. The results are discussed in evaluation section.
investigated evaluation protocols, but rather raising awareness in the community that we should carefully reconsider them in order to converge to their optimal usage.
As most of existing descriptors incorporate either color or spatial information; the proposed descriptor, called Grid-3CD, includes both information.
This descriptor is based on color connected components (CC) extracted from a quantified image.
It consists of a set of 6-tuples computed on a grid of pixels sampled from the color-quantified image. The 6-tuple of a given pixel describes the density, the mass center, the bounding box and the color of the CC that contains this pixel. The efficiency of this descriptor for identity document verification is shown using two strategies of pattern comparison. The first one is unsupervised and based on a distance measure whereas the second is supervised and based on one-class Support Vector Machine (SVM).
The experimentation of the new descriptor on four datasets of identity documents totaling 3250 documents shows an average accuracy of about 90%, outperforming state-of-the-art descriptors.
Results of experiments are presented to show the effect on the performance for: different ways of encoding the information, doing or not transfer learning and processing at text line or region level.
The results are comparable to the ones obtained on the ICDAR 2017 Information Extraction competition, even though the proposed technique does not use any dictionaries, language modeling or post processing.
This paper contributes to the field of Post-OCR Error Correction by introducing two Novel deep learning approaches to improve the accuracy of OCR systems, and a post processing technique that can further enhance the quality of the output results. These approaches are based on Neural Machine Translation and were motivated by the great success that deep learning introduced to the field of Natural Language Processing. Finally, we will compare the state-of-the-art approaches in Post-OCR Error Correction with the newly introduced systems and discuss the results.
We design a method to reuse layers trained on the IAM offline handwritten dataset to compute mid-level image representation for text in the Washington and Bentham dataset. We show that despite differences in the writing style, fonts etc. across these datasets, the transferred representation is able to capture a spatiotemporal representation leading to significantly improved recognition results. Additionally, we hypothesize that the performance is solely not dependent on the number of samples and our experimental evaluation tests the model with varying amount of fine-tuning samples showcasing promising results.
Short Papers Booklet
Poster Session I (Wednesday 25th April, 16:00-17:30)
Named Entity Recognition (NER), search, classification and tagging of names and name like frequent informational elements in texts, has become a standard information extraction procedure for textual data. NER has been applied to many types of texts and different types of entities: newspapers, fiction, historical records, persons, locations, chemical compounds, protein families, animals etc. Performance of a NER system is usually heavily genre and domain dependent. Entity categories used in NER may also vary. The most used set of named entity categories is usually some version of three partite categorization of locations, persons and organizations [1].
In our work we use a standard trainable statistical NER engine, Stanford NER . Considering the quality of our data and complexities of Finnish language, our NER results can be considered as good. With our ground truth data we achieve F-score of 0.89 with locations and 0.81 with persons. With re-OCRed Tesseract v. 3.04.01 output the F-score results are 0.79 and 0.72, respectively, for locations and persons.
Poster Session II (Thursday 26th April, 16:00-17:30)
character recognition requires a large number of training data since thousands of character classes exist in the language. In order to enhance Japanese scene character recognition, we have developed a training data augmentation method and a recognition system using multi-scale classifiers. Experimental results show that the multi-scale scheme effectively improves the recognition accuracy.
Demonstration
Attendee Information
You can enjoy some wine or beer at the welcome attendance which takes place at TUtheSky.
Registration Information
All participants need to register in order to attend the workshop. Admission to the workshop is not allowed without registration. Registration for the workshop, the tutorials and the social events is available online.
In order to correctly organize the workshop (social events, goodies, ...), the regular registration process will close after Monday 16th April 2018. The registration fee (both regular and student) includes admission to the workshop, coffee-breaks, lunch, USB proceedings, banquet and welcome reception.
In order to include the papers in the proceedings, at least one author of each paper must complete the registration form by February 16th, 2018. Student participants presenting more than one paper in the workshop are required to pay the regular registration fee (not student fee). According to IAPR's policy, should an author have more than one paper accepted, only one registration is required for publication although other authors are encouraged to register and participate in the workshop.
Each paper (oral or poster) must be presented by an author at the workshop. Failure to present a paper during the workshop will likely result in withdrawal of the paper from the conference digital library (Xplore).
Registration Fees
For early planning purposes, all attendees are encouraged to complete online registration before February 2, 2018, to enjoy the early bird discount registration fee.Student* | IAPR | Standard | |
---|---|---|---|
Early Bird** | 400€ | 550€ | 600€ |
Regular | 450€ | 600€ | 650€ |
* Master or PhD students
** until Feb 2, 2018
Social Events
The welcome reception takes place at TUtheSky (see picture above) which is in the heart of Vienna. The conference banquet will be held at the city hall (details will follow soon). Don’t miss the announcement of Best Paper and Best Student Paper winners and join your DAS colleagues for a dinner of wining, dining, and shining examples of research quality.
Discussion Groups
According to a long-standing tradition at the DAS workshops, we will hold small-group discussions on topics of special interest to attendees. It is a nice opportunity to meet other researchers and discuss on relevant topics for the community. Everyone is welcome to participate in the discussions. Moreover, each group needs a moderator and a scribe. Their roles are:
- The moderator encourages everyone to speak and helps to focus and clarify the discussion.
- The scribe takes written notes of the discussion and summarizes the results in a plenary session.
The moderator and the scribe will co-author a short summary report after the workshop is over, which will be posted to the workshop website and at the TC-11 website The names of the moderators and scribes will be also listed at the website.
Please fill this form choosing the topics of your interest, and feel free to propose some.
We are looking forward to your participation! Please contact the Discussion Groups coordinators with your suggestions and ideas:
Travel Information
If you fly to Vienna, you will arrive at Vienna International Airport (VIE), located at the city border about 20 minutes by public transportation. Conference attendees can take the City Airport Train (CAT), which directly connects the airport with the city. Alternatively you can take the bus (Vienna AirportLines) to Schwedenplatz or the railway S7. Vienna has a very dense and efficient public transportation network, and it is therefore easy to travel between the conference site, hotels and social venues; attendees will not need to rent a car. The network consists of five underground lines, trains, trams, and buses and runs around the clock. The central part of the city is relatively compact so you can reach most of Vienna’s attractions by foot.
Visa support letters
For those of you who require a visa support letter to attend the conference, please send us an e-mail: das2018@caa.tuwien.ac.at.
Austrian Airlines is Official Carrier of DAS 2018
If you book your flights to DAS 2018 online at www.austrian.com you can save 15% on all applicable fares. To do so, simply enter the following code in the eVoucher field on the Austrian homepage booking engine:
Code: DAS18
- Booking period: now until April 27th, 2018
- Valid for flights to Vienna and return as of April 17th, 2018 until Mai 4th, 2018 (final date for return flight) on flights operated by Austrian Airlines.
Book your flights here!
Travelling by train to Vienna
Vienna has direct connections to most European cities nearby, including Bratislava, Munich, Frankfurt, Budapest, Hamburg, Prague, Warsaw and overnight trains to Berlin, Venice, Rome, Warsaw and Zürich. Most long-distance trains run from the Hauptbahnhof (main station); many of these trains also serve Meidling. Some semi-fast services towards Salzburg start and end at Westbahnhof. Don’t confuse the main station (Hauptbahnhof) with the central station (Wien Mitte); the latter is only served by local and regional trains.
Travelling within Vienna
Vienna has an extensive public transport system (www.wienerlinien.at) that consists of five underground (U-Bahn) lines (U1, U2, U3, U4 and U6), trams and buses, which makes it easy to reach the conference venue, even if you are staying on the other side of the city. A single trip costs €2.20 and is valid on any reasonable route to your destination; changes are permitted. If you are going to spend time sightseeing or need to commute from your hotel, consider buying a pass. These are available for 24 hours (€7.60), 48 hours (€13.30) or 72 hours (€16.50). A weekly pass (€16.20) is even better value, but is only valid from Monday midnight (00:00hrs) to the following Monday, 9am. In common with many other European cities, Vienna’s public transport uses the honour system; the penalty for not having a valid ticket is €103. Note that many tickets require validation (stamping) before entering the platform; these are marked “Bitte entwerten/Please validate”.
Transfer from the airport to the city center
The Vienna International Airport (VIE) in Schwechat is about 20 km away in the southeast of Vienna. Taking a taxi directly at the airport is rather expensive (about €45), but you can get better value by pre-booking at airportdriver.at, flughafentaxi-wien.at (cost around €30), or myDriver.
Express trains (Railjet and Intercity) run at half-hourly intervals from the airport to the Hauptbahnhof (main railway station, line U1) and Meidling (U6) stations and take 15-18 minutes. If you are staying near the conference venue, you will probably want to use this service. A stopping service (S7) also runs across the city via Wien Mitte (Landstraße U3, U4), Praterstern (U1, U2) and Handelskai (U6), and connects with all underground lines; the travelling time to Wien Mitte is approx. 24 minutes. Regardless of which route you take, the fare within the integrated tariff system is €3.90 and this includes onward travel via subway, tram, bus, etc. to your destination in Vienna. If you already have a pass for Vienna, you need to purchase an extension ticket from the city boundary (ab Stadtgrenze, €2.20).
More information on the railway connections, including the timetable, is available in the following leaflet from the Austrial Federal Railways (ÖBB).
Premium services also include the City Airport Train (CAT, €12) and the Vienna Airport Lines buses (€8). The CAT runs non-stop to Wien Mitte, where it terminates; the main advantage is being able to check-in luggage at the railway station (select airlines only) on the day of your return flight. Departures are at 06 and 36 past the hour in both directions. Note that tickets purchased for these services are not valid for onward travel and are also not valid on the regular trains if you miss your connection.
Venue
Contact
TU Wien
Institute of Computer Aided Automation
Computer Vision Lab
Karlsplatz 13
A-1040 Vienna, Austria
Phone: +43-1-58801-18376
e-mail: das2018@cvl.tuwien.ac.at
Committee
General Chairs
Robert Sablatnig (Austria)
Florian Kleber (Austria)
Publicity Chairs
Markus Diem (Austria)
David Doermann (USA)
Gernot A. Fink (Germany)
Discussion Group Chairs
Alicia Fornés (Spain)
Marcus Liwicki (Germany)
Publication Chair
Stefan Pletschacher (UK)
Program Chairs
Basilis Gatos (Greece)
Koichi Kise (Japan)
Dan Lopresti (USA)
Jean-Marc Ogier (France)
Tutorial Chairs
Michael Blumenstein (Australia)
Stefan Fiel (Austria)
Cheng-Lin Liu (China)
Program Committee
Adel Alimi (Germany)
Apostolos Antonacopoulos (UK)
Oliver Augereau (Japan)
Elisa H. Barney Smith (USA)
Abdel Belaid (France)
Vincent Christlein (Germany)
Hervé Déjean (France)
Andreas Dengel (Germany)
Rafael Dueire Lins (Brazil)
Véronique Eglin (France)
Jihad El-Sana (Israel)
Andreas Fischer (Switzerland)
Volkmar Frinken (USA)
Utpal Garain (India)
Lluis Gomez (Spain)
Venu Govindaraju (USA)
Masakazu Iwamura (Japan)
Motoi Iwata (Japan)
Dimosthenis Karatzas (Spain)
Bart Lamiroy (France)
Laurence Likforman-Sulem (France)
Josep Lladós (Spain)
George Louloudis (Greece)
Andreas Maier (Germany)
R. Manmatha (USA)
Simone Marinai (Italy)
Program Committee (continued)
Jean-Luc Meunier (France)
Guenter Muehlberger (Austria)
Masaki Nakagawa (Japan)
Premkumar Natarajan (USA)
Umapada Pal (India)
Shivakumara Palaiahnakote (Malaysia)
Thierry Paquet (France)
Vincent Poulain D'Andecy (France)
Ioannis Pratikakis (Greece)
Jean-Yves Ramel (France)
Oriol Ramos Terrades (Spain)
Marcal Rusinol (Spain)
Joan Andreu Sanchez (Spain)
Marc-Peter Schambach (Germany)
Srirangaraj Setlur (USA)
Faisal Shafait (Pakistan)
Fotini Simistira (Switzerland)
Nikolaos Stamatopoulos (Greece)
Karl Tombre (France)
Alejandro Toselli (Spain)
Seiichi Uchida (Japan)
Berrin Yanikoglu (Turkey)
Mauricio Villegas (Turkey)
Berrin Yanikoglu (Turkey)
Konstantinos Zagoris (Greece)
Richard Zanibbi (USA)
Sponsors